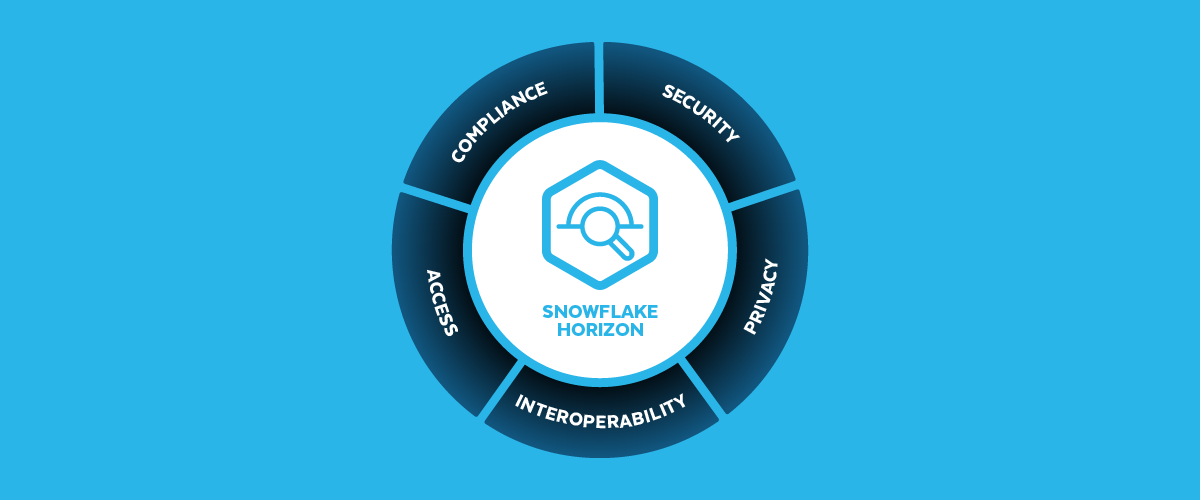
Video
Snowflake Horizon Demo for Data Governors And Stewards
In this demo targeted at data governors and stewards, learn and try the core capabilities of Snowflake Horizon such as classifying and tagging sensitive data, visualizing lineage, monitoring data quality, and applying granular policies.